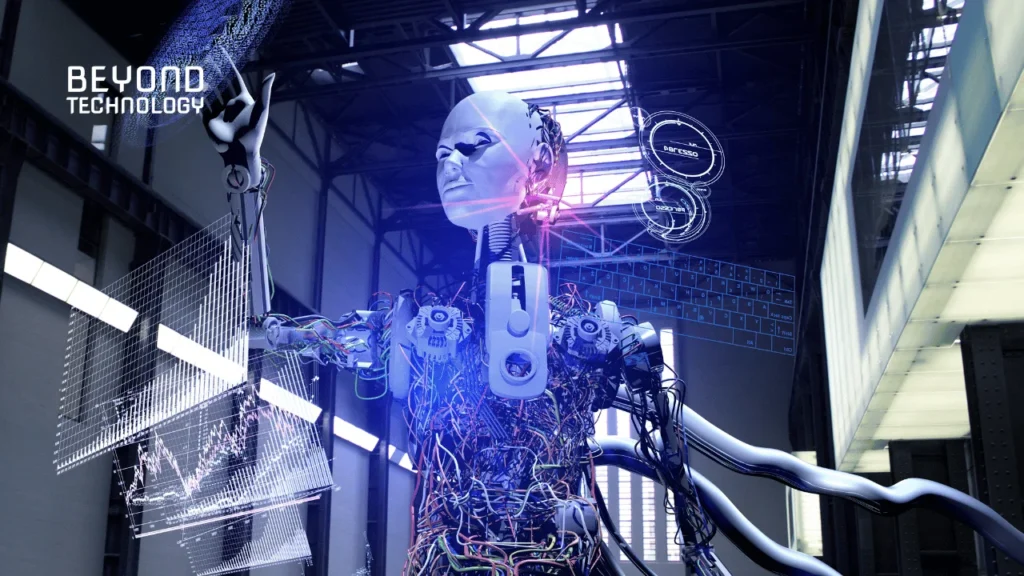
Artificial Intelligence (AI) is revolutionizing countless sectors, and predictive analytics is no exception. In this article, we will explore in depth how artificial intelligence is transforming predictive analytics, from the fundamentals to practical applications and future challenges.
What is Predictive Analytics?
Predictive analytics uses statistical techniques, algorithms, and machine learning models to analyze historical data and make predictions about future events. It is a branch of advanced analytics that enables organizations to anticipate trends, behaviors, and outcomes, thus improving decision-making.
Key Components of Predictive Analytics
- Data: The foundation of any predictive model. Data can come from various sources, including internal databases, social media, IoT sensors, etc.
- Models: Use mathematical and statistical algorithms to identify patterns in the data.
- Validation and Evaluation: Models are tested and evaluated to ensure their accuracy and reliability.
- Implementation: Predictive models are integrated into enterprise systems to provide real-time insights.
The Role of Artificial Intelligence in Predictive Analytics
Artificial intelligence, especially machine learning and deep learning, has taken predictive analytics to new levels of precision and applicability. Let’s see how:
Machine Learning
Machine learning allows predictive analytics models to learn from data and improve over time without human intervention. Machine learning algorithms are used to uncover hidden patterns in large volumes of data, providing more precise and actionable predictions.
Key Machine Learning Algorithms
- Linear and Logistic Regression: Used to predict continuous values and event probabilities.
- Decision Trees and Random Forests: Allow data segmentation and prediction based on multiple variables.
- Support Vector Machines (SVM): Effective for classification and regression in complex problems.
- Neural Networks: Mimic the functioning of the human brain to recognize complex patterns and make predictions.
Deep Learning
Deep learning, a subset of machine learning, uses artificial neural networks with multiple layers (deep neural networks) to model complex relationships in the data. It is particularly useful in applications such as image recognition, natural language processing, and real-time prediction.
Convolutional Neural Networks (CNN)
CNNs are particularly effective for analyzing visual data. They are used in the detection of anomalies in medical images, object identification in photographs, and the automation of computer vision tasks.
Recurrent Neural Networks (RNN)
RNNs are ideal for processing sequential data, such as time series and text analysis. They are used in applications such as financial forecasting, sentiment analysis, and text generation.
Applications of Artificial Intelligence in Predictive Analytics
The integration of artificial intelligence in predictive analytics has opened up a wide range of applications across various sectors. Below are some notable examples:
Healthcare
- Diagnosis and Personalized Treatment: Predictive models can analyze patient data to anticipate diseases and personalize treatments.
- Epidemic Forecasting: AI can predict disease outbreaks and aid in response planning.
Finance
- Credit Risk Prediction: AI models can assess the creditworthiness of clients and predict defaults.
- Investment Optimization: Predictive analytics can identify market trends and optimize investment portfolios.
Retail
- Inventory Management: AI can forecast product demand and optimize inventory.
- Offer Personalization: Predictive models can analyze customer behavior and personalize product recommendations.
Manufacturing
- Predictive Maintenance: AI can predict equipment failures and plan maintenance before a breakdown occurs.
- Production Optimization: Predictive analytics can improve production efficiency and reduce costs.
Challenges and Considerations
Despite the advances, the integration of artificial intelligence in predictive analytics faces several challenges:
Data Quality
The accuracy of predictive models depends heavily on data quality. Incomplete, incorrect, or biased data can lead to erroneous predictions. It is crucial to implement robust data management practices to ensure the integrity and accuracy of the data used.
Model Interpretability
AI models, especially those based on deep learning, are often considered black boxes due to their complexity. The lack of interpretability can be an obstacle to their adoption in sectors where transparency and explainability are critical. Techniques such as LIME (Local Interpretable Model-agnostic Explanations) and SHAP (SHapley Additive exPlanations) are being developed to address this issue.
Privacy and Security
The use of large volumes of personal data raises concerns about privacy and security. It is essential to comply with regulations such as GDPR (General Data Protection Regulation) and CCPA (California Consumer Privacy Act) to protect individuals’ sensitive information.
Integration and Scalability
Integrating AI-based predictive models into existing systems can be complex and costly. Additionally, the scalability of these models to handle large volumes of data and make real-time predictions requires advanced technological infrastructures.
The Future of Artificial Intelligence in Predictive Analytics
The future of artificial intelligence in predictive analytics is promising. Here are some emerging trends and developments that will likely shape the landscape in the coming years:
Hybrid Models
Hybrid models that combine machine learning techniques with traditional approaches are gaining popularity. These models can offer the best of both worlds, providing accurate and explainable predictions.
Quantum computing has the potential to revolutionize predictive analytics by solving complex problems much faster than classical computers. Although still in its early stages, advancements in this area could open new possibilities for data analysis and prediction.
Explainable Artificial Intelligence (XAI)
Research in explainable artificial intelligence is accelerating, aiming to make AI models more transparent and understandable. This is crucial for their adoption in regulated sectors and for gaining user trust.
Integration of IoT and Big Data
Combining artificial intelligence with the Internet of Things (IoT) and Big Data will enable the creation of more robust and real-time predictive systems. The ability to analyze real-time data from connected sensors and devices will provide more accurate and timely insights.
Model Automation
The development of AutoML (Automated Machine Learning) platforms is simplifying the creation and optimization of predictive models. These platforms allow users with no data science expertise to build effective AI models, democratizing access to advanced predictive analytics.
Artificial intelligence is transforming predictive analytics, taking it to new levels of precision, efficiency, and applicability. From machine learning to deep learning, AI technologies are enabling organizations to anticipate and respond to future events with unprecedented accuracy. However, to fully leverage these opportunities, it is essential to address the challenges related to data quality, model interpretability, privacy, and technological integration.